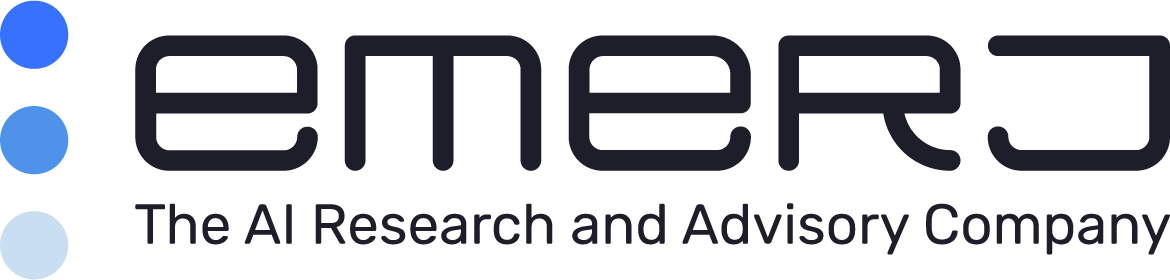
Solving AI Hallucinations
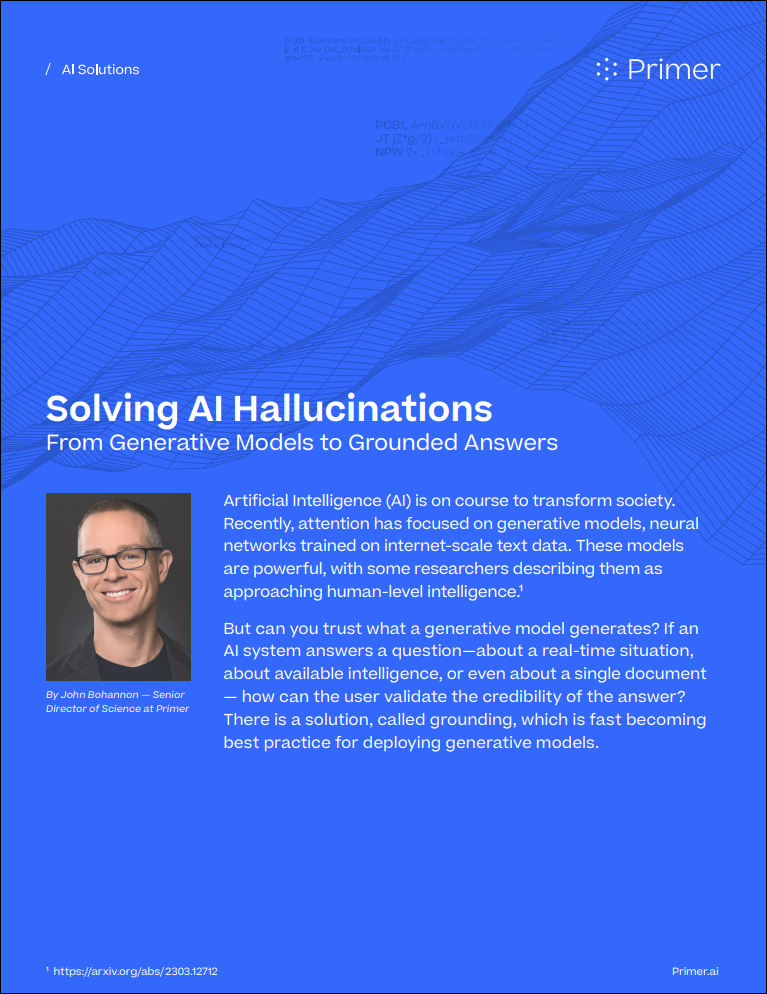
From Generative Models to Grounded Answers
For intelligence analysts and military operators bearing the responsibility of safeguarding lives, reliability is a critical feature in AI systems. Large Language Models (LLMs) are incredible engineering tools, capable of automating repetitive workflows, speeding up intelligence cycles, and helping deciders make more informed decisions faster. But if not designed correctly, these models can generate text that is confident, articulate, and yet factually incorrect.
In this whitepaper, Dr. John Bohannon will delve into the concept of “grounding” and its potential as a solution to mitigate hallucinations in LLMs. Dr. Bohannon will not only explore the mechanics of grounding but will also shed light on the broader challenges faced by organizations on a path to AI-driven transformation. With insightful explanations and real-world examples, readers will gain practical insights on how to strike a balance between embracing the potential of innovative technology like LLMs and addressing their limitations.
In this white paper, you’ll gain insight on:
- How hallucinations happen
- Solving hallucinations through “grounding”
- RHLF and Instruction-tuning
A clear, sober, non-technical explanation of how generative AI models operation and how the training phase of their development leads to misappropriated context and unreliable responses.
Introducing a novel approach to model development by using “grounded” to retrieve relevant information from a trusted system of record and provide genAI models with appropriate prompts for higher accuracy in answers.
Providing leaders with a ‘deeper dive’ into the fundamentals of RHLF and new approaches to fine-tuning in the model development process that rely on ‘instructive’ data sets with rigorous standards for replies.
Created by: